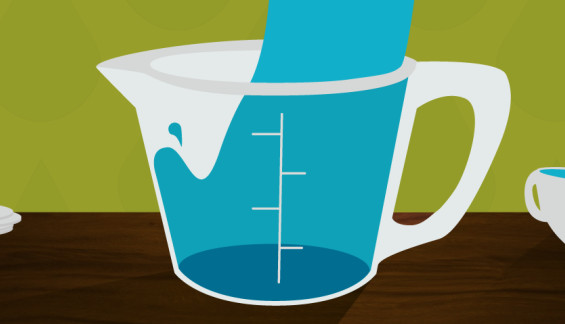
Measuring changes in water consumption can be a tricky business. Most homes in the United States have only a single water meter that is read bi-monthly. This makes it challenging to determine changes in consumption patterns from period to period, or to identify what approaches to encouraging water-use efficiency are actually most effective.
As a water utility manager, this makes it hard to decide how to invest limited resources to improve operational efficiency, extend asset lifetimes, or better engage with customers. This is where the scientific method, and more specifically, scientific control, comes into play. The idea behind scientific control is to run an experiment that identifies the effect of a single (or independent) variable on a treatment group in comparison to a group that does not receive treatment (the control group). If the only difference between the treatment group and the control group is the independent variable, then any observed differences between the two groups can be attributed to the variable. The purpose of this scientific control is to determine causation, rather than to just note a correlation. We want to know if the independent variable is actually responsible for the difference between the two groups.
For a clearly ridiculous illustration of correlation, examine the data below that plots the highway fatality rate in the United States with the tons of lemons imported from Mexico during the same period.
No rational person would argue that lemon imports cause highway fatalities. While the data are correlated, there is no causation in this example. This correlation is, in essence, a coincidence.
In order to prove causation, a statistically valid experiment would need to be performed that controlled for lemon imports (the independent variable) and tracked highway fatalities over time. While this is an impractical experiment to perform, it illustrates how correlation can be used to mislead. This is why a statistically significant controlled experiment is so important to determine causation. And in order to perform such an experiment, two sufficiently large groups (typically great than 1,000) need to be randomly selected with one group being ‘treated’ and the other being left alone as a control.
So how does this relate to water efficiency? Many utility efficiency programs are based on allowing customers to volunteer, or opt-in, to participate. In many cases, those customers who choose to participate in an efficiency program do see greater levels of water savings than those users who don’t choose to participate. But this is simply a correlation. It may be that users who choose to participate would be more likely to save to begin with, and it’s their temperament, cultural background, political persuasion, income or some other factor that leads to savings, not participation in a program.
In order to prove that a program yields results, a proper scientific experiment must be conducted. A randomly selected group of users must be selected as a treatment group and be enrolled into an efficiency program on an ‘opt-out’ basis. This means that they will be included in the program unless they explicitly decline. Another randomly selected group will be assigned as the control. By choosing two, randomly assigned groups, we are able to control for demographic or other factors that might impact savings behavior. The only statistically significant difference between the two groups is the enrollment in the efficiency program. Therefore, any differences in savings between the treatment group and the control group can be entirely attributed to the program (the independent variable).
While this all may sound quite wonky, in reality it is actually very important. If a water utility is truly serious about better managing its resources, it must make investment decisions based on sound quantitative data. Simply assuming a correlation is an inadequate basis for an investment decision.
“If a water utiltiy is truly serious about better managing its resources, it must make investment decisions based on sound quantitative data.”
If you were told that the stock price for Coca-Cola was correlated with a growth in the stork population, would you buy Coca-Cola stock when there was a spate of baby stork births? Of course not. For just this reason, utilities should not be investing in programs that are correlated with water savings. Any program worth its salt will incorporate an opt-out program design and controlled experiment that can validate and quantify savings, thus providing the water utility with the data it needs to make better-informed investment decisions in the future. This will yield greater savings, and lower costs for customers over time. Don’t confuse correlation with causation, otherwise it may result in money flowing down the drain.